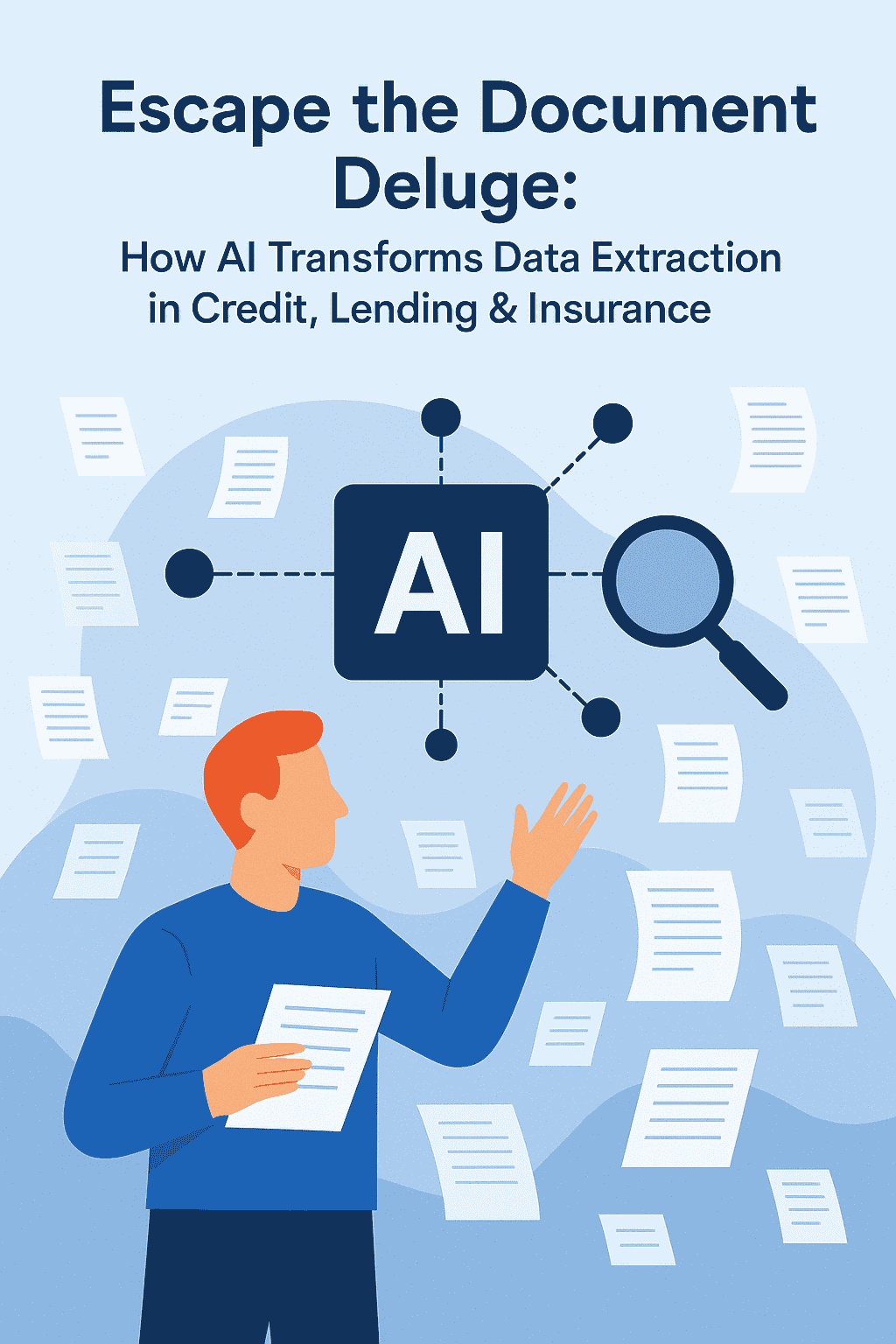
Escape the Document Deluge: How AI Transforms Data Extraction in Credit, Lending & Insurance
Introduction: Drowning in Documents? There’s a Smarter Way Forward
The financial services and insurance (FSI) sectors – encompassing banking, lending, and insurance – are fundamentally built on information. This information, critical for assessing risk, processing transactions, and serving customers, overwhelmingly resides within documents. Loan applications, bank statements, credit reports, insurance claims, policy declarations, underwriting submissions, financial statements, contracts – the list is extensive and ever-growing. As digital transformation accelerates, the sheer volume of this data, often in digital formats like PDFs and emails, is increasing exponentially.
However, simply having these documents isn’t enough. The real challenge lies in extracting the valuable data trapped within them. For decades, this has largely been a manual process – teams of skilled professionals painstakingly reading through pages, identifying key information, and keying it into various systems. This traditional approach isn’t just tedious; it’s a significant, often underestimated, drain on resources, efficiency, and accuracy. The reliance on manual handling introduces substantial risks, from costly errors and compliance failures to fraud vulnerabilities and slow customer service.
But there’s a lifeline. Artificial Intelligence (AI), particularly advanced technologies like Large Language Models (LLMs), offers a revolutionary way forward. These tools go beyond simple scanning; they can understand the content and context of documents, intelligently extracting critical data points with unprecedented speed and accuracy. The core issue isn’t merely the volume of documents, but the fact that a vast majority—often estimated at over 80%—of enterprise data is unstructured, residing in formats like emails, PDFs, scanned images, and free-text notes. Traditional automation tools and basic Optical Character Recognition (OCR) struggle immensely with this lack of structure, creating operational bottlenecks. AI, specifically LLMs, is uniquely equipped to bridge this gap by interpreting meaning and context within these varied formats. Furthermore, the shift towards digital processes hasn’t eliminated the document challenge; it has often merely changed the medium from paper to digital files like PDFs or emails, which still require manual interpretation or extraction. Digitization alone is not the solution; intelligent understanding is.
This post delves into the realities of manual document processing in FSI, explores how AI and LLMs provide a powerful alternative, and explains how tailored solutions, like those from exathinklabs, offer a balanced approach combining automation with essential human oversight. Readers will gain a clear understanding of:
- The true, quantified cost of sticking with manual processes.
- How AI and LLMs work for document extraction, explained in plain English.
- The power of fine-tuning AI for specific FSI needs.
- The tangible benefits (speed, cost, accuracy) supported by real numbers.
- How a guided approach ensures accuracy and compliance in AI adoption.
The Crushing Weight of Manual Document Processing in FSI
For professionals in credit risk, lending, and insurance operations, the daily reality often involves navigating a sea of documents. Manually reading, identifying, cross-referencing, and keying in data from diverse sources like loan applications, financial statements, claims forms, and policy documents is a core part of the job. This work is not only repetitive and time-consuming but also represents low-value activity that diverts skilled personnel from more strategic tasks like complex analysis, customer interaction, or risk mitigation.
The time commitment is staggering. Claims associates, for instance, can spend up to 80% more time on manual data entry compared to their counterparts using automated processes. Processing a substantial volume, like 100,000 pages of documents annually, can consume approximately 5,000 person-hours, translating to a potential cost of $250,000 based on an hourly rate of $50. Specific processes suffer significant delays: manually processing a single invoice takes an average of 14.6 days, and the typical mortgage origination process stretches across 35 to 40 days, largely due to manual document verification. Research indicates that 56% of companies dedicate over 10 hours per week solely to manual invoice handling.
Beyond the hours logged, the direct financial costs are substantial. Manual invoice processing can cost anywhere from $12 to $35 per invoice, with labor accounting for roughly 62% of these expenses. In the insurance sector, the cost of manual rework averages $25 per claim, highlighting the financial burden of correcting inevitable errors. For financial institutions, onboarding a new customer through processes involving document processing and Know Your Customer (KYC) verification can cost between $150 and $300, with manual handling of exceptions contributing 30-50% of that cost. Compounding these direct costs are indirect financial drains, such as missed early payment discounts or incurred late fees resulting from sluggish processing cycles, and potentially lost business due to delays and subpar customer experiences.
Perhaps even more concerning than the time and cost is the inherent inaccuracy of manual processes. Human error is unavoidable, especially in repetitive data entry tasks. Studies show that manual data entry errors occur in 1% to 4% of all transactions. In insurance, error rates in manual claim assessments range from 5% to 12%, leading to costly underpayments or overpayments. Astonishingly, 39% of invoices are found to contain errors, contributing to problems like duplicate payments. The consequences of these errors are severe, leading to flawed risk assessments, incorrect insurance premium pricing, erroneous loan approvals, and ultimately, poor business decisions based on faulty data. Nearly 70% of finance professionals admit to making critical decisions based on financial data they know might be inaccurate due to manual processing limitations.
The negative impacts ripple throughout the organization. Manual processes create significant operational bottlenecks, especially during peak periods like insurance renewals or mortgage refinancing waves, severely limiting scalability and responsiveness. For a majority of financial institutions (67%), document processing remains their most significant operational bottleneck. This inefficiency extends to compliance and risk management. Managing sensitive data securely through manual workflows is challenging, increasing the vulnerability to compliance violations and hefty fines – FINRA, for example, has imposed over $90 million annually for violations, including improper insurance policy management. Furthermore, manual processes are fertile ground for fraud; businesses relying on manual accounts payable controls experience an 80-90% increase in fraud cases, and the insurance industry faces over $80 billion in fraudulent claims payouts annually. Finally, the customer bears the brunt of these inefficiencies through slow service (like auto repair claims taking over 23 days post-pandemic), lack of transparency, and errors, leading to frustration, distrust, and potential customer churn.
It becomes clear that manual document processing isn’t merely inefficient; it actively increases exposure across multiple critical risk vectors. The financial risks stem from errors, rework, and fraud. The operational risks manifest as bottlenecks and scalability issues. Compliance risks arise from potential data mismanagement and failure to meet regulatory standards. Reputational risks emerge from poor customer experiences. This multi-faceted vulnerability underscores the systemic weakness inherent in relying solely on manual methods. Moreover, while the direct labor costs are undeniably high, the downstream financial impact of inaccuracy—encompassing rework, bad debt from poor lending decisions, fraud losses, compliance penalties, and lost business opportunities—likely dwarfs the payroll expenses associated with manual processing. This reframes the value proposition: investing in accuracy is not just about saving labor costs, but about mitigating potentially far larger financial damages.
Table 1: The True Cost of Manual Document Handling in FSI
Key Metric | Traditional Manual Process |
---|---|
Avg. Processing Time | • Invoice: 14.6 days • Mortgage: 35-40 days • Claim (Auto Repair): 23.1 days • Per Page: ~3 minutes |
Avg. Cost per Item | • Invoice: $12 - $35 • Insurance Rework: $25 per claim • New Customer Onboarding (Doc/KYC): $150 - $300 |
Typical Error Rate | • Data Entry Transactions: 1-4% • Insurance Assessments: 5-12% • Invoices with Errors: 39% |
Annual Fraud Cost Exposure (Insurance) | USD $80 Billion+ |
Compliance Risk Factor | High - Penalties (e.g., FINRA >$90M/yr), Data Management Challenges |
Staff Time on Manual Entry (vs. Automated) | Up to 80% more time |
Operational Bottleneck Rank (FIs) | #1 for 67% of institutions |
AI & LLMs: Your Intelligent Document Decoding Team
For years, the primary technology for digitizing documents was Optical Character Recognition (OCR). While useful, OCR simply converts an image of text into machine-readable characters. It doesn’t understand the meaning, context, or structure of the information it’s converting. Think of OCR as a basic transcription service – it captures the words, but doesn’t grasp their significance.
Intelligent Document Processing (IDP) represents a significant leap forward. Powered by Artificial Intelligence (AI), IDP solutions go beyond simple text recognition. They can automatically classify different document types (like distinguishing an invoice from a loan agreement), extract specific pieces of data (like names, dates, amounts, policy numbers), and crucially, understand the context in which that data appears. Extending the analogy, if OCR is a transcriber, IDP/AI is like an intelligent assistant who not only transcribes but also comprehends the discussion, identifies key action items, extracts relevant facts, and organizes the information meaningfully.
At the heart of many advanced IDP systems lies a powerful type of AI known as Large Language Models (LLMs). These are sophisticated AI models trained on truly massive datasets – billions of web pages, books, articles, and other text sources. This extensive training allows them to develop a deep understanding of human language, including grammar, nuances, context, and the intricate relationships between words and concepts.
LLMs are particularly adept at handling the unstructured data that plagues FSI workflows – information found in emails, free-form text fields in applications, scanned correspondence, legal contracts, and detailed reports. This is precisely where traditional, rule-based automation systems falter, as they rely on predictable structures and formats. LLMs, however, can make sense of this variability, unlocking insights from data sources that were previously difficult or impossible to process automatically. Imagine an LLM as an incredibly well-read subject matter expert who has absorbed the content of countless documents. It doesn’t just recognize keywords; it grasps the underlying concepts, understands definitions, and perceives how information connects, even when presented differently across various document types.
How do LLMs achieve this for data extraction?
- Deep Contextual Understanding: Unlike keyword searching, LLMs analyze the surrounding text to interpret meaning. They can understand that “Gross Monthly Income” on a pay stub is conceptually equivalent to “Applicant Salary” on a loan form, even if the wording differs. In a complex insurance policy, an LLM can differentiate between the policyholder, beneficiaries, coverage limits, and exclusions because it understands the roles and relationships described in the text.
- Format and Layout Flexibility: Because they focus on semantic meaning rather than fixed locations, LLMs can extract data from documents with varying layouts and formats (PDFs, Word files, scanned images, emails) without needing rigid, pre-defined templates for each variation. This adaptability is crucial in FSI, where document standards can differ significantly.
- Practical Examples:
- Lending/Credit Risk: An LLM can process a bundle of documents for a loan application, including multiple bank statements, tax returns, and credit reports. It can identify and extract recurring income sources, calculate debt-to-income ratios, flag potentially risky large cash deposits or withdrawals, and summarize the applicant’s financial health in a structured format suitable for input into a credit decisioning engine.
- Insurance Claims: When an insurance claim is submitted, often with a mix of structured forms and unstructured documents like adjuster’s notes, police reports, repair estimates, or medical narratives, an LLM can intelligently scan the entire package. It can extract key details like policyholder information, date and nature of the incident, specific damages claimed, associated costs, and identify any inconsistencies or missing documentation required for processing.
Furthermore, LLMs possess capabilities like Zero-Shot and Few-Shot Learning. This means that due to their vast pre-training, they can often perform new data extraction tasks accurately with very few examples (few-shot) or sometimes even with just a clear instruction (a “prompt”) and no specific examples (zero-shot). This significantly accelerates the deployment of AI for new document types or extraction needs, reducing the time and data required for training.
The true advantage of LLMs in FSI document processing, therefore, stems from their capacity for semantic understanding and contextual interpretation, moving far beyond simple text recognition. This capability unlocks the immense value hidden within the vast quantities of unstructured data prevalent in the sector. It signifies a fundamental shift away from brittle, rule-based or template-dependent automation systems, which often break when encountering variations in document formats, towards adaptive, learning-based systems that can handle real-world document diversity much more effectively.
Fine-Tuning: Training Your AI for Peak FSI Performance
While general-purpose Large Language Models (LLMs) possess remarkable capabilities thanks to their training on vast, diverse datasets, achieving optimal performance for the specific, high-stakes tasks within the financial services and insurance industries often requires an additional step: fine-tuning.
Fine-tuning is essentially a process of specialized training. It involves taking a powerful, pre-trained LLM – one that already has a broad understanding of language and the world – and further training it on a smaller, curated dataset that is highly specific to the target industry or task. Think of it like taking a brilliant, highly educated university graduate (the pre-trained LLM) and enrolling them in a specialized FSI certification program. This targeted training equips them with the specific jargon, regulations, document types, and analytical nuances required to excel as a credit analyst, insurance underwriter, or claims specialist.
Why is this specialization so critical for credit, lending, and insurance?
- Mastering Industry Jargon: FSI is replete with specialized terminology, acronyms, and complex contractual language (e.g., Loan-to-Value (LTV), Debt Service Coverage Ratio (DSCR), subrogation clauses, policy endorsements, ACORD forms, regulatory codes like IFRS 9). A general LLM might struggle to interpret these terms correctly in all contexts. Fine-tuning on FSI-specific documents helps the AI master this unique vocabulary, leading to significantly higher accuracy in data extraction and interpretation. Some specialized solutions are trained on tens of thousands of industry-specific terms.
- Handling Specific Document Types: FSI relies on numerous unique document formats – loan applications, mortgage disclosures, insurance policy schedules, claims forms (like ACORD), loss run reports, reinsurance treaties, financial prospectuses, KYC documents, etc.. Fine-tuning allows the AI to become proficient at navigating the specific layouts, structures, and data fields common to these documents, even when variations exist.
- Boosting Task-Specific Accuracy: For critical FSI tasks – accurately extracting complex financial figures from statements, identifying subtle risk indicators in underwriting reports, verifying data against compliance checklists, classifying different types of insurance claims, or detecting potential fraud patterns – fine-tuning sharpens the AI’s focus and improves its reliability.
- Efficiency and Cost-Effectiveness: Building an FSI-specific LLM from the ground up would require immense computational resources, vast amounts of specialized data, and prohibitive costs. Fine-tuning leverages the billions of parameters and knowledge already embedded in the pre-trained model, making it a much faster, more economical way to achieve high performance on specialized tasks.
- Mitigating Bias and Enhancing Control: General LLMs trained on broad internet data may inadvertently learn biases present in that data. Fine-tuning on carefully selected, relevant FSI datasets provides an opportunity to guide the model’s behavior, reduce the risk of generating biased or inappropriate outputs, and align it more closely with ethical and regulatory standards.
The result of fine-tuning is an AI system that is not only intelligent in a general sense but also highly proficient and reliable within the specific context of financial services and insurance. It becomes a more accurate, contextually aware digital assistant tailored to the industry’s unique demands, risks, and regulatory landscape. This specialization is essential for building trust and ensuring dependable performance in an environment where errors can have significant financial and reputational consequences. Fine-tuning effectively bridges the gap between the broad capabilities of general AI and the precise, high-stakes requirements of regulated sectors like FSI, making advanced AI practical and trustworthy for critical operations. Furthermore, the ability to adapt existing powerful models through fine-tuning democratizes access to sophisticated AI, allowing FSI institutions beyond the largest tech companies to develop and deploy tailored AI solutions.
Unlocking Efficiency: The Measurable Impact of AI Document Automation
The transition from manual document handling to AI-powered Intelligent Document Processing (IDP) delivers transformative results that can be measured across multiple dimensions. The statistics paint a compelling picture of efficiency gains, cost reductions, and accuracy improvements.
Dramatic Speed Enhancements: AI automation drastically accelerates workflows that were previously measured in days or weeks. Document processing times can be slashed by 50% to over 90% in many cases. Manual claims processing, which could take days or even weeks (with auto repair claims averaging over 23 days), can often be reduced to hours or minutes with AI. Similarly, loan processing times can be cut by 50% to 70%. The lengthy mortgage origination process, typically 35-40 days manually, sees significant reduction, with potential cuts of up to 80% in document processing time cited. Invoice processing, averaging over two weeks manually, can be completed in seconds or minutes. In one case, a bank reduced new account onboarding time from 24 days to just 3 days using advanced document processing.
Significant Cost Savings: These speed improvements translate directly into substantial cost reductions. Operational costs associated with document processing can be lowered by 20% to as much as 80%. Labor savings are a major contributor, with automation potentially reducing manual data entry effort by 70% or more. One financial services company reported saving $2.9 million annually by reducing its manual document extraction team size after adopting IDP. The return on investment (ROI) for IDP implementations is often rapid, with studies showing 30% to 200% ROI within the first year, primarily from labor cost savings. Some estimates suggest payback periods of just 12-18 months for mortgage document intelligence systems.
Quantum Leaps in Accuracy: AI systems significantly reduce the errors inherent in manual data entry and interpretation. Error rates can be decreased by over 52%. Advanced IDP systems, particularly those leveraging fine-tuned LLMs, can achieve data extraction accuracy rates exceeding 99%. Specific applications show remarkable precision, such as detecting fake paystubs with 99.8% accuracy in loan processing. This high accuracy minimizes the need for costly rework and corrections.
Increased Capacity and Throughput: Automation allows organizations to handle significantly higher volumes of work without proportionally increasing staff. Insurers have reported processing 200 more forms per day. Accounts payable departments using AI-driven IDP have increased invoice processing throughput by as much as 60% compared to manual methods. In one documented case, an engineering firm using IDP managed to process 400% more requests for proposals (RFPs). Some insurers leveraging AI platforms have even quadrupled their overall operational capacity for handling submissions.
These impressive metrics translate directly into tangible business value for FSI organizations:
- Faster Decision-Making: Quicker loan approvals, accelerated claims settlements, and speedier underwriting translate into a superior customer experience and a significant competitive edge in time-sensitive markets. Insurers have reported an 85% improvement in speed-to-quote.
- Reduced Risk Profile: Lower error rates mean fewer costly mistakes like incorrect claim payouts or improperly assessed loans. Enhanced data analysis capabilities improve fraud detection, identifying suspicious activities earlier and more effectively. Automation also aids in maintaining consistent compliance with regulations.
- Enhanced Customer Satisfaction: Faster response times, fewer errors requiring correction, and smoother overall processes lead to happier, more satisfied customers, fostering loyalty and reducing churn.
- Strong Return on Investment: The combination of substantial cost savings, efficiency gains, risk reduction, and improved customer retention delivers compelling ROI, often recouping the initial investment quickly.
The benefits are clearly interconnected, creating a virtuous cycle. Faster processing lowers costs and improves the customer experience. Reduced errors minimize financial losses and compliance risks. Increased capacity allows for growth without proportional increases in overhead. Freed-up staff can focus on higher-value activities like complex analysis, strategic initiatives, and personalized customer service, further enhancing business performance. The sheer scale of these improvements—often involving reductions of 50%, 70%, or even 90% in time and cost, coupled with accuracy approaching 100%—indicates that AI document automation is not merely an incremental upgrade; it represents a fundamental transformation of core, document-intensive workflows within the FSI sector.
Table 2: AI/IDP Transformation: Before vs. After in FSI
Key Metric | Traditional Manual Process | AI-Powered Process (IDP/LLM) |
---|---|---|
Avg. Processing Time (Loan/Claim) | Days to Weeks (e.g., Mortgage: 35-40 days, Invoice: 14.6 days) | Hours to Minutes (50-90%+ reduction, 80% cut in mortgage doc time possible) |
Cost per Document Processed | High (e.g., Invoice: $12-$35, Rework: $25/claim) | Significantly Lower (Operational costs reduced 20-80%) |
Data Accuracy Rate | Lower (Error rates 1-12%) | High (Up to 99%+, Errors reduced >52%) |
Staff Time on Manual Entry | High (Up to 80% more time than automated, >10 hrs/week on invoices) | Minimal (Manual effort reduced 60-80%+) |
Throughput Capacity | Limited by manual labor | Significantly Increased (e.g., +60% invoice throughput, 4x operational capacity) |
Exathinklabs: Intelligent Automation with Essential Human Oversight
While the potential of AI and LLMs to automate document processing in FSI is immense, the high-stakes nature of the industry—involving significant financial transactions, sensitive customer data, and strict regulatory oversight—demands more than just raw automation. Accuracy, reliability, compliance, and trust are paramount. This is where Exathinklabs positions itself not merely as a technology provider, but as a partner enabling trusted and responsible AI adoption.
Central to the Exathinklabs approach is the concept of the “Guided AI Credit Risk Associate”. This framework embodies the principle of Human-in-the-Loop (HITL), integrating essential human expertise and oversight into the automated workflow. In practice, this means AI handles the heavy lifting – ingesting documents, classifying them, extracting data, performing initial analyses, and flagging potential issues or exceptions. However, critical decision points, complex cases, high-value transactions, or instances where the AI expresses low confidence are routed to human experts for review and final judgment. These experts – seasoned underwriters, experienced credit risk analysts, skilled claims adjusters – leverage the AI’s output but apply their domain knowledge, intuition, and contextual understanding to validate findings and make the ultimate call. The AI acts as a powerful assistant, augmenting human capabilities rather than completely replacing them.
Crucially, the Exathinklabs solution incorporates robust monitoring and validation mechanisms. Recognizing that AI models, particularly LLMs, operate probabilistically, continuous monitoring of AI performance and validation of extracted data against predefined rules or external sources ensures ongoing accuracy and reliability. This systematic oversight builds confidence in the automated processes.
This hybrid, human-guided approach offers distinct advantages specifically tailored for the FSI sector:
- Balancing Speed with Trust: It captures the significant efficiency gains of AI automation while retaining the critical layer of human judgment necessary for high-stakes financial decisions. This fosters trust in the technology among users, management, and regulators.
- Handling Complexity and Exceptions: AI excels at processing routine tasks and identifying patterns in large datasets. Humans, however, are better equipped to handle novel situations, ambiguous information, or complex edge cases that fall outside the AI’s training data. HITL ensures these exceptions are managed correctly.
- Ensuring Compliance and Explainability: In heavily regulated industries like finance and insurance, the ability to explain why a decision was made is critical. Human oversight ensures that automated processes adhere to regulatory requirements (like fair lending practices or claims handling standards) and that decisions derived from AI outputs can be justified to auditors and regulators. This directly addresses the “black box” problem often associated with complex AI models.
- Facilitating Continuous Improvement: The feedback provided by human experts when they review or correct AI outputs serves as valuable data for further refining and improving the AI models over time. This creates a continuous learning loop, making the AI progressively smarter and more accurate.
- Promoting Ethical AI: Human involvement provides a crucial check against potential biases that may exist in AI models or the data they were trained on. This oversight helps ensure fairness and equity in decision-making processes, particularly in sensitive areas like credit lending.
- Facilitating Continuous Improvement: The feedback provided by human experts when they review or correct AI outputs serves as valuable data for further refining and improving the AI models over time. This creates a continuous learning loop, making the AI progressively smarter and more accurate.
- Promoting Ethical AI: Human involvement provides a crucial check against potential biases that may exist in AI models or the data they were trained on. This oversight helps ensure fairness and equity in decision-making processes, particularly in sensitive areas like credit lending.
The Exathinklabs “Guided AI Associate” model delivers the best of both worlds. It maximizes the transformative efficiency and cost-saving benefits of AI automation while embedding the control, accuracy, validation, and compliance safeguards essential for the FSI sector. The focus shifts from mere task replacement to intelligent augmentation, empowering FSI professionals by equipping them with powerful tools. For FSI, this balanced approach isn’t just preferable; it’s arguably the only responsible and sustainable path to leveraging AI effectively. This HITL component is non-negotiable for building the trust required for deploying AI in core operational workflows. Furthermore, by automating the monotonous aspects of document processing, this model elevates the role of FSI professionals, allowing them to transition from manual data entry clerks to strategic analysts, validators, and decision-makers – roles that leverage their expertise and likely lead to greater job satisfaction and organizational value.
Conclusion: Embrace Intelligent Automation, Guided by Expertise
The evidence is clear: relying on traditional, manual methods for document processing in the credit risk, lending, and insurance sectors is becoming increasingly unsustainable. The sheer volume of documents, coupled with the high costs, inherent inaccuracies, significant risks, and operational bottlenecks associated with manual handling, presents a formidable challenge. Sticking with the status quo means accepting inefficiency, elevated fraud and compliance risks, and potentially falling behind competitors who embrace smarter solutions.
Artificial Intelligence, particularly the advent of Large Language Models (LLMs) and the ability to fine-tune them for specific industry needs, offers a powerful and transformative solution. These technologies move beyond simple digitization to provide genuine understanding, enabling the intelligent extraction of critical data from even complex, unstructured documents with remarkable speed and accuracy. The measurable impact—drastic reductions in processing time and operational costs, significant improvements in data accuracy, enhanced risk management, and increased capacity—demonstrates that AI is not just an incremental improvement but a fundamental shift in how FSI organizations can operate. The market for Intelligent Document Processing is growing rapidly, with projected market sizes reaching tens of billions of dollars within the next decade, reflecting widespread adoption across industries, led significantly by BFSI.
However, for industries built on trust and subject to stringent regulation, deploying AI requires careful consideration. Exathinklabs recognizes this imperative, offering a “Guided AI Associate” approach that strategically integrates human expertise with AI automation. This monitored, validated, human-in-the-loop model ensures that organizations can harness the power of AI responsibly, maintaining the accuracy, compliance, and explainability crucial for financial services and insurance. It provides a reliable and trustworthy pathway to AI adoption, augmenting the capabilities of skilled professionals rather than simply automating tasks.
The time for contemplation is over. FSI organizations need to actively explore and adopt intelligent automation to remain competitive, manage risk effectively, and deliver the seamless experiences customers now expect. Embracing AI, guided by essential human expertise, is no longer just an option – it’s a strategic necessity for navigating the future of finance and insurance.
Ready to Transform Your Workflow?
Schedule a demo to see how ExaThinkLabs can streamline your document analysis process.
Schedule a personalized demo today to see how Exathinklabs can help you escape the document deluge and unlock new levels of efficiency and accuracy.